GPU-enhanced cloud services leverage the power of Graphics Processing Units (GPUs) to deliver high-performance computing in a cloud-based environment. These services are particularly beneficial for machine learning tasks, offering superior parallel processing and the capacity to handle large-scale data operations.
In a B2B context, these cloud services play a critical role in monitoring machine learning models. Proper monitoring ensures that these models remain accurate and reliable over time, which is crucial for businesses that rely on machine learning for data-driven decisions. The ability to track, evaluate, and fine-tune models in real-time helps B2B organizations maintain a competitive edge in a rapidly evolving landscape. By utilizing GPU-enhanced cloud services, companies can efficiently monitor their machine learning models and ensure optimal performance.
Monitoring is a crucial aspect of maintaining effective machine learning models. It ensures that models continue to deliver accurate and reliable results over time, which is essential for businesses that depend on these models for decision-making and operational efficiency. Without proper monitoring, models can drift or degrade, leading to incorrect predictions and potentially significant business risks.
Effective monitoring helps detect issues such as data drift, where changes in input data can affect a model's accuracy, and concept drift, where the underlying relationships in the data change over time. By keeping track of these factors, businesses can take corrective action to recalibrate or retrain models as needed.
The Nebius GPU cloud provider offers high-performance cloud-based infrastructure designed for monitoring machine learning models. It provides the computational power needed for real-time analytics and anomaly detection, enabling businesses to ensure their models remain accurate and reliable. Proper monitoring also contributes to enhanced performance, as it allows companies to identify and address bottlenecks or inefficiencies in their machine learning workflows.
Key Features of GPU-Enhanced Cloud Services for Monitoring
GPU-enhanced cloud services offer a range of features that make them ideal for monitoring machine learning models. One of the most notable features is real-time data processing, allowing businesses to track and analyze model outputs as they occur. This capability is crucial for identifying trends and spotting anomalies quickly.
Parallel computing is another key feature. GPUs excel at performing multiple computations simultaneously, enabling these cloud services to handle complex monitoring tasks efficiently. This is particularly useful for B2B companies that need to monitor multiple models or large datasets in real-time.
High computational capacity is also a significant advantage. GPU-enhanced cloud services provide ample processing power to run intensive machine learning workloads while monitoring them concurrently. This capacity ensures that businesses can maintain high levels of performance and accuracy, even as their monitoring needs grow.
Techniques for Monitoring Machine Learning Models
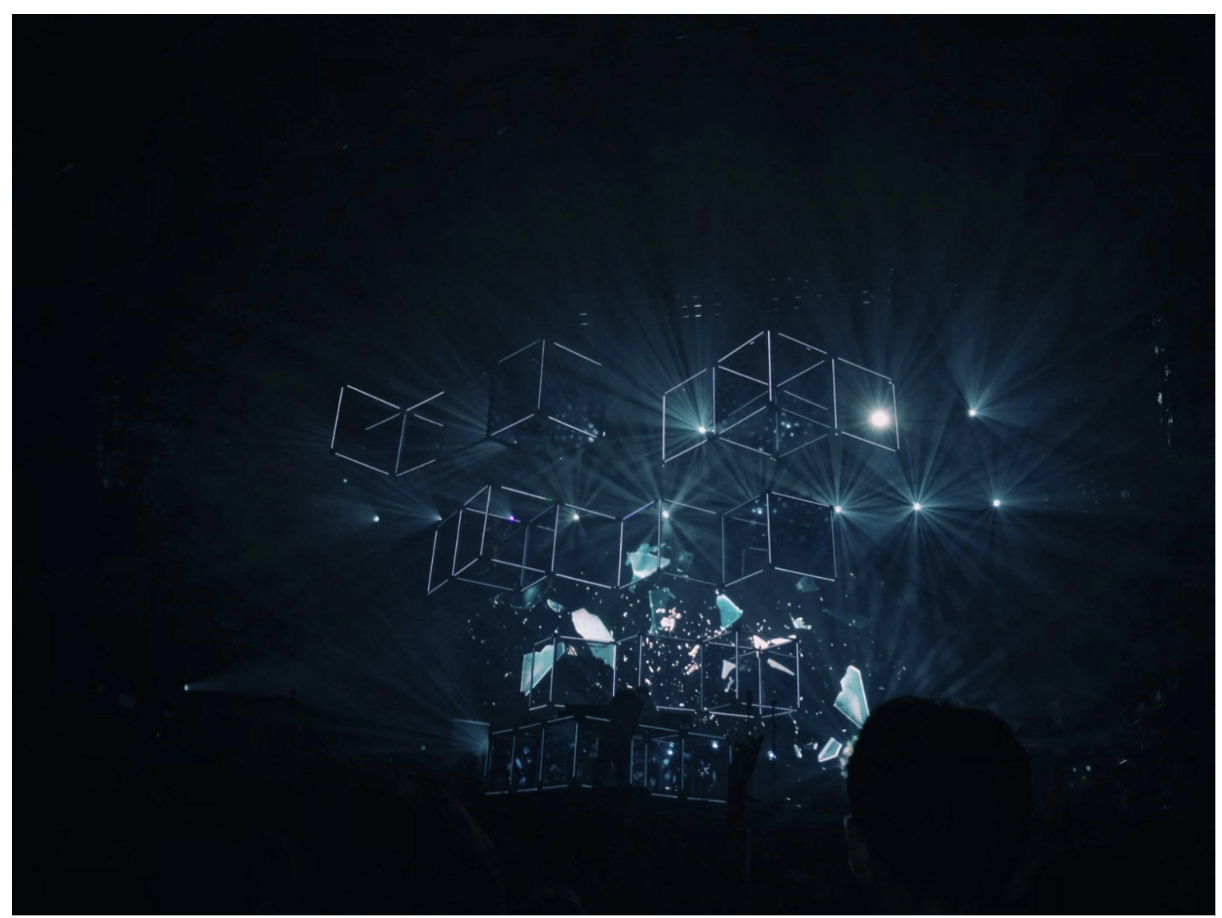
Various techniques can be used to monitor machine learning models with GPU-enhanced cloud services. Real-time analytics is a common approach, allowing businesses to analyze model outputs as they are generated. This technique helps detect issues like data drift and concept drift early, enabling prompt corrective actions.
Anomaly detection is another valuable technique. By using GPU-enhanced cloud services, businesses can leverage sophisticated algorithms to identify outliers or unexpected behavior in their models. This helps ensure that models remain reliable and do not produce erroneous results.
Performance tracking is also crucial for monitoring machine learning models. GPU-enhanced cloud services provide the resources needed to track key metrics such as response times, throughput, and resource utilization. By monitoring these metrics, businesses can optimize their models for better efficiency and address any performance bottlenecks.
These techniques, enabled by the high computational power of GPU-enhanced cloud services, contribute to robust monitoring practices that help maintain the accuracy and reliability of machine learning models.
Benefits of Using GPU-Enhanced Cloud Services for Monitoring
GPU-enhanced cloud services offer numerous benefits for monitoring machine learning models. A significant advantage is scalability. These cloud services can easily scale resources to meet changing monitoring demands, providing flexibility for businesses with varying workloads. This scalability is crucial for B2B companies that need to adjust their monitoring capacity based on business needs.
Flexibility is another key benefit. GPU-enhanced cloud services allow businesses to customize their monitoring environments, offering a range of hardware configurations and software options. This flexibility enables companies to tailor their monitoring setup to specific machine learning tasks, enhancing efficiency and performance.
Cost-efficiency also plays a vital role. By leveraging the scalability of GPU-enhanced cloud services, businesses can optimize resource utilization and reduce costs. Pay-as-you-go and other flexible pricing models allow companies to manage expenses effectively, making these services a cost-efficient solution for monitoring machine learning models.
Challenges and Solutions in Monitoring with GPU-Enhanced Cloud Services
Monitoring machine learning models comes with unique challenges. A common issue is data drift, where shifts in data patterns can affect model accuracy. GPU-enhanced cloud services address this with real-time analytics and anomaly detection, enabling businesses to quickly spot and correct such changes.
Another challenge is handling peak workloads. GPU-enhanced cloud services offer auto-scaling to dynamically adjust resources based on demand, ensuring smooth operation even during high-traffic periods. Load balancing is also essential, distributing tasks across multiple GPUs to prevent bottlenecks and maintain optimal performance.
Data redundancy is crucial for monitoring, providing backup solutions in case of hardware failure or other disruptions. By replicating data across different locations, GPU-enhanced cloud services ensure business continuity and reduce the risk of data loss.
These solutions help B2B companies overcome the challenges of monitoring machine learning models, ensuring consistent performance and reliability.
Security and Compliance in Monitoring Machine Learning Models
Security is a critical concern when monitoring machine learning models. GPU-enhanced cloud services implement robust security measures to protect sensitive data. Data encryption is a key feature, ensuring that information remains secure during storage and transmission. This level of encryption helps prevent unauthorized access and data breaches.
Access controls are also important, allowing businesses to define who can access specific resources. This feature helps maintain data integrity and limits the risk of unauthorized use.
Compliance with industry regulations is another vital aspect. GPU-enhanced cloud services adhere to standards such as GDPR and HIPAA, ensuring that businesses meet legal requirements for data protection. Compliance is essential for maintaining customer trust and avoiding penalties.
Conclusion
GPU-enhanced cloud services offer a scalable and flexible solution for monitoring machine learning models. With features like real-time processing, auto-scaling, and robust security, these services are poised to play a significant role in the future of B2B machine learning. As AI becomes more integral to business operations, GPU-enhanced cloud services will continue to drive innovation and ensure the reliable monitoring of machine learning models.